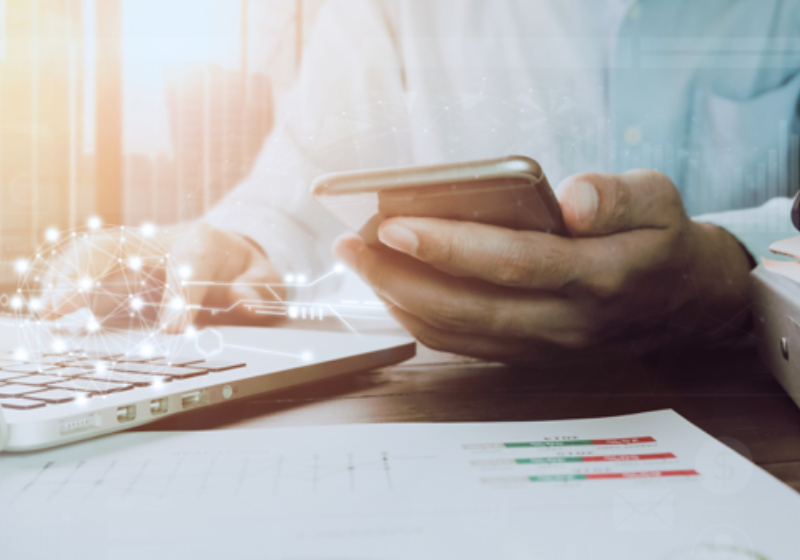
By Tim Holt 26 min read
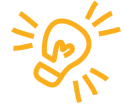
1. What is ‘data quality’?
Your business collects a high number of data from different sources each day. Each source of data is a business asset that, when stored and processed in a high-quality environment, can help drive strategic decision making and marketing campaigns.
What are your most common sources of business data?
- Client and prospect business data and contact details
- Customer and partner interactions and enquiries
- Business transactions
- Leads and pipeline information
- Social media audience data
- Employee information, skills, performance review and contracts
- Product SKU specs and service information
- Business correspondence
- Process details such as order fulfilment, customer support and disaster recovery plans
- Inventory details and supply chain data
- Risk management data
- Profit and loss data, month end reports and annual financial statements
- Market size, competitor pricing and market share
Companies that utilise a greater number of internal and external data sources have the advantage of conducting a wider and more granular range of data analyses for marketing and business strategies.
TIP: Good data governance, data quality, as well as accessibility and usability of information, become more important as your data sources increase.
Data quality, therefore, is essential for growing your business. Incorrect business decisions could be taken if your data is inaccurate. With high-quality data, improved targeting will ensure you get the right message to the right person, increasing the likelihood of conversions and sales.
So, can good quality data drive down your overall business costs?
Yes! Here are a few examples:
- Quality data allows you to be more efficient; the right message gets to the right audience and increases your ROI.
- With low quality data you waste time reconciling data issues and resolving anomalies and errors.
- Reduced marketing spend and increased sales have a positive impact on P&L.
- Quality data enables you to cross sell an additional product or service to an existing customer
- Better data gives companies the ability to effectively sell add-ons and upgrades they may be interested in.
- Better customer insights improve customer retention
- More insight on your customers helps you react faster to any new product development opportunities.
Read more about how high quality data can grow your business in our blog post.
With businesses gathering data from more and more locations, there’s potential for data quality and governance problems, especially when data originates from external sources, or purchased from untrustworthy third parties.
At Data HQ, our ongoing process of research and data updates indicates that the number of business contacts moving or changing roles is increasing year on year, which is particularly evident in the current difficult economic environment.
But, if you’re at risk of buying, holding or processing ‘bad data’, you first need to know what ‘bad data’ is.
What makes bad data?
Bad data refers to information that can be erroneous, misleading, and without general formatting. Unfortunately, no industry or organisation is immune to it. If not acknowledged and fixed early on, bad data can cause serious strategic or customer relation issues.
If you’re buying B2B data, you need to be aware of the quality of the data your purchasing.
The first risk associated with buying cheap data is the quality. Cheap data lists are riddled with problems usually including a high level of inaccuracy, a significant number of duplicate records and no real targeting in terms of matching the audience to your requirements. These reasons alone can make cheap data a false economy.
As well as the money lost to the purchase, there is a tangible risk to a business’ reputation when using poor quality, cheap data. It can result in miscommunication with customers, spammed contacts and ineffective targeting.
Using bad data can have serious knock on consequences to your brand equity. Read more about the dangers of buying cheap B2B data in our blog.
Secondly, it must be remembered that even quality data decays incredibly quickly.
Our own telephone research indicated that, in a 12 month period:
- 38.9% of the contacts in the 20,000 largest UK companies have either left the business or changed position.
- 6.32% of HR and 16.06% of IT contacts have left or changed roles.
- 28.82% of marketing and 29.76% of sales personnel have left or changed positions.
Keeping your in-house customer and prospect data clean, therefore, is of the utmost importance. By using accurate and clean data, your business intelligence will be more insightful, and your sales and marketing campaigns will be more successful.
Think your database is clean? Read more about the dangers of data decay in our blog, here today gone tomorrow.
2. Key data quality metrics
Data Quality (DQ) Dimensions are recognised terms used by data professionals to describe measurable or quantifiable features against defined standards to determine the quality.
The most common data quality metrics are completeness, accuracy, consistency, validity, timeliness and integrity.
Below are their definitions, and alternative terms for the same metrics.
Dimension | Definition |
Completeness | Completeness measures the degree of population of data values in a data set. Are data values missing, or unusable? In some cases, missing data is irrelevant, but when the information that is missing is critical to a specific business process, completeness becomes an issue. |
Accuracy | Accuracy measures the degree to which data factually represents its associated real-world object, event, concept or alternatively matches the agreed upon source(s). Incorrect spellings of business or personal names, addresses, and even untimely or not current data can impact operational and analytical applications. |
Consistency | Consistency measures whether or not data is equivalent across systems or location of storage. Are values consistent across data sets? Inconsistency between data values plagues organizations attempting to reconcile between different systems and applications. |
Validity | Validity measures whether a value conforms to a preset standard. Is the data presented in the correct and pre-defined format, type or range so as to be applicable to the given analytical task? |
Timeliness | Timeliness is a measure of time between when data is expected versus made available. How long is the time difference between data capture and the real world event being captured? |
Integrity | Integrity measures the structural or relational quality of data sets. The inability to link related records together may introduce duplication across your systems. The inability to link related data instance together impedes this valuable analysis. |
Failings in any of these dimensions can compromise the usefulness of your data. Let’s look at each in turn.
How can you ensure your data meets the required standards?
It is advisable to halt your marketing and communications project if you recognise that your data is not accurate or complete. Next:
- When collecting data organically, make more use of mandatory fields function, data completeness is easily achievable. The respondent will not be able to complete and submit the data without the mandatory fields being filled. This will also ensure less time being wasted fixing mistakes resulting from incomplete data.
- Use drop down menus over free text fields in a data collection application; this will result in data that is consistently collected in the expected format.
- Try to use real-time data as often as possible, older forms of data can be subject to decay. For marketing lists, when real-time information is almost impossible, ensure the data is as up-to-date as possible to minimise decay.
TIP: To ensure you don’t buy incomplete data, go back to your provider and find out how often they update their list. To maintain the quality of their mailing lists, data providers should have a robust data verification process in place. The verification process could be manual or automatic, but the outcome should be the same: high quality, accurate data on real prospects.
There are, of course, many suppliers who build, source and maintain high quality data lists of both consumer and business contacts.
The sources of the data within these legitimate lists include:
- Companies House, Directories, Credit Reference agencies, Publishers and Event companies for B2B data
- Electoral Roll, BT OSIS, Publishers, Events, Form Completion, Subscriptions and Surveys for B2C contact lists.
Good suppliers will also make sure the data gathered to compile their lists is run through the appropriate suppression files such as Gone Aways, Deceased, Change of Address and Business Changes files.
Read more about the importance of data quality metrics, and the signs of good and bad data in our blog post.
3. Why is high quality data valuable?
“Fortune 1000 enterprises will lose more money in operational inefficiency due to data quality issues than they will spend on data warehouse and customer relationship management (CRM) initiatives.”
Gartner
According to a Senior Analyst at Forrester, “Just a 10% increase in data accessibility will result in more than $65 million additional net income for a typical Fortune 1000 company.”
Dirty data can cause a wide array of problems for businesses, in all aspects of the revenue cycle. What’s more, because of cheap, low quality data suppliers, bad data is creeping into our marketing automation and CRM systems. The impact varies from a transactional loss to widespread catastrophe on an enterprise.
Did you know, according to a study by FastMap and Consumer Intelligence, just 35% of organisations have a regular data cleansing process in place?
According to Halo Bi, The implementation of a data quality initiative can lead to reductions of:
- 10-20% corporate budget
- 40-50% IT budget
- 40% operating costs
And increases of:
- 15-20% in revenue
- 20-40% more sales
What’s wrong with buying cheap data?
Cheap data is riddled with problems — a high level of inaccuracy, a significant number of duplicate records and no real targeting in terms of matching the audience to your requirements.
Some of the implications of cheap, low-quality data include:
- Poor targeting and micro-targeting
- Irrelevant creative and messaging, even wrong or inappropriate
- Inappropriate or incorrect levels of personalisation
- Wrong-time media delivery
- Wasted media pounds
- Risk to brand affinity, intent, purchase and loyalty
- Lack of competitive differentiation
Poor quality data can also lead to wasted budget in postal and telemarketing campaigns, with the funds being spent on ineffectual print, postage and packaging or the staffing costs of conducting a telemarketing campaign.
According to Experian Quality Data, the average company wastes 12% of revenue due to inaccurate information in their records.
TIP: Be wary of a data supplier that is quick to drastically cut the price of their data without an understanding of why you may require a lower rate. Credible suppliers are, on the whole, open to negotiation but would expect to understand the business reasons behind the requirement for cheaper marketing data
Ultimately, dirty data can lead to lost revenue, wasted marketing efforts, misinformed decisions and, eventually, damaged reputation.
How can you improve your ROI from data? Improve your data quality.
With better quality data, companies can:
- Determine which components of their service propositions are resonating with their ideal customers, and which are not.
- Accurately measure database marketing campaigns for impact and effectiveness.
- Better understand their customer lifetime value, which allows them to identify their most profitable customer segments.
- Identify business areas of opportunity and pinpoint those opportunities that can add the most value.
What are the warning signs that your data is too cheap? Read them in our blog.
Did you know, data quality improves user satisfaction?
From a customer point of view, having quality data allows you to adopt a more personalised approach. Receiving relevant, tailored communications will resonate more with the recipient increasing their perception / satisfaction.
From an employee point of view, quality data will reduce frustration. Contact rates will be higher both via phone and email plus lower levels of returned postage. All in all this will reduce friction within the business.
At Data HQ we believe the better the data quality the better the decision making within the business. Accurate data will deliver better results, reduce business risk and boost profit.
There are many ways that quality data drives value. Read more about how high quality data can grow your business in our blog post.
Why choose a quality data provider?
Whilst your own quality business data is highly valuable, so is a trusted, high quality third party data provider. With uncertainty about the data quality on offer, however, buying a marketing database is not an easy decision.
TIP: As a bonus question, ask the company what action they will take if a message turns out to be undeliverable. Their answer may tell you a lot about the quality of their service.
There are a few important questions to ask your third party provider to ensure you’ll get high quality data before you make any purchases. These include:
- How current is your data?
- What deliverability guarantees do you have?
- How often are numbers screened against the CTPS register?
- Are you DMA members?
- Where do you obtain their data?
- What else can you do with your data?
To ensure your own business data quality, it’s essential to choose a third party data provider that matches your own quality processes. To find out more, read our blog outlining what to look for in a data provider.
4. Getting your data quality in order
Before you can start cleaning your data, preventing dirty data, and enhancing your data, you need to understand the overall state of your data. Ask yourself, how dirty is your database?
If you don’t know, you need to assess and benchmark your data quality.
TIP: Subsequent to your own audit (or an external audit) you may decide to undertake a cleansing process to improve your files’ data quality. Alternatively you may have discovered your data is well-maintained internally and does not require updating.
The most obvious benefit from cleansing is the money that can be saved by not mailing to inaccurate customer or prospect details, as well as protecting and improving your brand perception by generating more accurate communications.
Going forward, a vital first step to maintaining data quality is ensuring that your data entry systems are effective and are used efficiently by those working with them every day, such as:
- Sales team entering client and sales details
- Marketing department monitoring response rates
- Accounts team entering financials
- Customer service personnel compiling feedback
- Engineers reporting on equipment or faults
- Shop staff monitoring promotion uptakes.
Data management, cleansing and processing
You should first begin evaluating the quality of your data with a data assessment.
TIP: Many vendors offer a free assessment to help identify areas where data quality can be improved, what types of data may be missing, and other problems that may be affecting optimal data performance.
Getting your data cleansed will help keep your campaign costs down and thereby improve your ROI. You can start this process yourself by:
- Undertaking some in-house management and cleansing;
- Ensuring consistent formatting for data entry;
- Making clear the importance of precise and accurate data entry to any staff entering the information;
- Combining and de-duping files based on email address or another unique reference within the files.
Following this initial activity, you may need the assistance of a data-marketing specialist or provider, like Data HQ, to help with the more advanced stages of the processing and cleansing of your data.
They will be able to:
- Identify, remove, or consolidate remaining duplicate records;
- Suppress or update data that is no longer valid.
Sometimes, it’s a good idea to create your own data quality assessment checklist. An example can be seen below:
Category | ✔ | ✘ | Comments |
| |||
Is there a direct relationship between the activity and what is being measured? | |||
Is data collected appropriately and with GDPR compliance? | |||
Are the staff collecting data qualified and supervised? | |||
Are steps being taken to correct known data errors? | |||
Are known data collection problems appropriately assessed? | |||
Are there steps to limit transcription error? | |||
Are data quality problems clearly described in final reports? | |||
2. Completeness | |||
Is there a method for detecting duplicate data? | |||
Is there a method for detecting missing data? | |||
3. Accuracy | |||
Are there proper safeguards in place to prevent unauthorised changes to the data? | |||
Is there a need for an independent review of results reported? | |||
4. Consistency | |||
Is a consistent data collection process used from year to year, data source to data source? | |||
Are there procedures in place for periodic review of data collection, maintenance and documented in writing? | |||
5. Timeliness | |||
Is a regularized schedule of data collection in place to meet program management needs? | |||
Is data properly stored and readily available? | |||
Summary | |||
Based on the assessment relative to the five standards, what is the overall conclusion regarding the quality of the data? | |||
Actions needed to address limitations |
Carrying out an audit will provide a clear vision of the current situation. The benefits include:
- Identifying out-of-date information for removal
- Highlighting available enhancements for your current database
- Saving budget and improve customer service
- Avoiding contacting customers who are no longer appropriate
- Improving response rates and ROI
Ready to find out more about the quality of your database? Read more about why a data audit is essential to ensure the quality of your data in our blog.
Ultimately, the most important aspect of managing your data quality is to be proactive not reactive.
According to Experian, there are four stages of data quality sophistication:
Unaware
Some businesses are unaware of the importance of their data quality and its impact on their business. In these organisations, incomplete and inaccurate data is seen as sufficient and minimally reactive processes are implemented when needed.
Reactive
These organisations react to data quality issues as they impact business performance, but there is limited sophistication when dealing with data at a corporate level. Investments in data quality are typically in response to specific, compelling issues in the short term, like a compliance breach.
Proactive
These organisations have clear, proactive data management strategies, with defined roles and responsibilities around data within the business. Data processes are better understood across departments, and silos have begun to be broken down, allowing for collaboration between IT and business users.
Optimised
In some cases, organisations have developed a fully governed data quality environment, where the data quality of their marketing database is monitored with documented with full sets of rules in place.
There is a single owner responsible for corporate-wide data management, consolidating their processes and technology investment.
You should aim to be a proactive or optimised business to ensure your data quality processes are robust, and your business is not losing key revenue to bad data.
Find out more about the process of cleansing your data and avoiding wasting budgets in our blog.
5. How to supplement existing data
There’s two aspects to data quality — ensuring the data you collect or buy is accurate and optimised. At the same time, you want to ensure that your older, existing data is used to the best of its capability.
As well as collecting and buying data, it is likely that your organisation already manages a variety of data systems such as:
- Sales / CRM system
- Supply chain management
- Accounting systems
- Marketing leads
To get the most value from this data, to enhance your marketing campaigns and bring in more sales, you need to enhance your existing data sources. These enhancement include:
- Creating a Single Customer View
- Customer segmentation
- Identifying data gaps
- Updating your database
What is a Single Customer View?
Many businesses struggle to segment and personalise their communications for their individual customers. Building a Single Customer View (SCV) allows all the data you hold about each of your customers to be stored and consolidated into one single, easy to read record in your database.
With only a partial view of your customers, your understanding of their true value is limited. You could miss opportunities, risk contradictory reporting, and potentially alienate customers if you are limited to a “one size fits all” marketing strategy.
TIP: Creating a single version of the truth, giving consistent results, can power everything from campaign management to data analysis, customer insight and business decision-making.
Maintaining and effectively using the data within your systems is a huge step towards a successful SCV. Then, consolidating your customer data, ensuring you’re not storing duplicate or disparate data, vastly improves targeting, personalisation and removes guesswork.
How is a SCV achieved?
- Use specialist tools to allow access, cleanse and enhance source data.
- De-duplicate and merge data sources into the single view.
- Flag data by source, maintaining its original integrity along with the enhanced, consolidated version.
Read more about creating and maintaining a Single Customer View in our blog: SCV — the complete story.
Create specific customer segments
With a clean, SCV database in place, you can use analytics to identify, communicate and target customers with the most relevant offers.
Begin by creating customer segments. Customer segmentation refers to dividing customers into groups who share similar characteristics, such as age, gender, lifestyle, and so on.
How to segment your customers
Any number of segments can be created, but it is important to ensure that your segments are:
- Not too narrow — the segment should be worthy of marketing efforts and not cost-prohibitive
- Accessible — your segment of consumers must contactable through established channels
There are many ways to segment your audience data; the most common include:
- Geography
Segment your audience by their postcode, county, or other geographic factor; useful if you are promoting a local service or a regional event.
- Age
Your products or services may be better suited to a specific age group; being specific with your messages for each group will help improve campaign results.
- Gender
Are your products more male or female-oriented? Consider if you should change your messaging to appeal more closely to different genders/
- Industry
Consider which industries your products are designed for, or if you want to target different industries to grow your market share.
- Job role
Your messaging for different job roles may need vary substantially.
- Hobbies
Segmenting by individuals’ interests will allow you to communicate the relevant promotions or content to your segments.
- Order history
Some clients order low volumes or low value items monthly, whereas others order high-end products every 4-6 months. Segmenting these groups will allow you to target appropriate value and types of products.
- Stage of buying cycle
Understanding where customers sit in the buying process will enable you to automate and personalise your introduction, cross-selling, up-selling and ongoing engagement messages.
Read more about why segmentation can improve your relationship with your customers, and enhance your Single Customer View in our blog — how to best segment your audience.
Consider where there might be data gaps.
Once you’ve cleaned and segmented your audience, you may find there will be areas you can or have previously identified as gaps, where you feel information would benefit the business.
Uncovering these gaps could require additional analysis or augmenting your existing data with external data sources or research.
TIP: When enhancing your database with a third party, ensure your provider has discussed and fully understands your business.
How can you supplement your data?
If you identify gaps in your database or customer profiles, you can enhance your information with a number of standard business variables.
For example, data can be enhanced and maximised by standardising the address format and using a Postal Address File (PAF).
In general, a basic profile can be enhanced with the following data fields:
- 2003 Digit SIC Code/Description
- Industry Code/Description
- Employee Band
- Turnover Band
- Location Indicator - Head Office, Single Site & Branch
If you’re unsure how to enhance your existing data or build a single customer view, consider working with a third party data provider like Data HQ.
TIP: A trusted provider will build you a tailor made Single Customer View for your business, communicating directly with all your data source systems, as well as data mining and marketing automation tools where required.
What happens when you have your data cleansed by a data solutions provider like Data HQ?
- A third party provider will match your list to their own database or ‘universe’ – allowing them to append information where the information you hold varies from their universe.
- Their data universe will have a guaranteed level of recency – so potentially it will be more up to date than your own. Their knowledge and expertise in data will also assist in standardising the format of the data you hold.
- They will screen your data against a number of industry-standard suppression files to ensure that old, out-of-date data is removed.
- They can sometimes provide a new address for existing customers who may have moved without informing you. Often, this can be an invaluable source of new data and a method of reactivating lapsed customers.
- A data provider will discuss any remaining requirement for combining files and further de-duplication as required, to finalise the process.
Read more about why it’s important to segment and manage your database in our blog — stay in control – manage multiple data sources.
6. Protecting the quality of a new database
GDPR has upped the data quality ante for businesses storing customer data — its implementation on 25 May 2018 will impact those companies holding poor-quality customer data, such as incomplete, out-of-date or duplicate records.
- Under the GDPR, any organisation found to be using inaccurate customer data without a recognised legal basis, such as consent, could face a significant fine.
- Where failure to have a strong foundation of good, accurate data could be a risk, the GDPR also requires organisations to implement measures to mitigate this risk.
Other GDPR requirements such as consent, data security and governance are often viewed in isolation but all these requirements rely on good data. It’s important therefore not to overlook data quality to focus on just these aspects.
Involve your staff and stakeholders
Unfortunately, it is not uncommon for data quality to become an afterthought, with staff reacting to errors rather than proactively rooting out the causes of data flaws.
A key element in any new database quality project, therefore, is the early involvement and understanding of key staff and stakeholders within your business. This might include directors, sales teams and others. There will be specifics you need your staff to do when gathering or inputting data, to ensure their output is suitable to work into your new combined database.
TIP: Having the right people in place to champion your data quality practices is essential to creating a business culture that supports data quality as status quo.
Why should you standardise data entry?
Often, data standardisation is left out of discussions across departments when outlining the processing of company data, especially when inputting leads into a CRM system.
Without standardisation, different iterations of your data will exist and be committed to your CRM, causing inconsistent, incomplete or missing data, outdated information and duplicates. Enforcing data standardisation processes ensures:
- An organised and consistent environment for entering and managing.
- Data can be shared more easily across your enterprise, establishing trust and reducing silos.
Ideally, standardisation should be performed during data entry. If, for any reason this is not possible, a comprehensive backend process is necessary to eliminate any inconsistencies.
What about human error?
A vital first step to maintaining data quality is ensuring that your data entry systems are effective and are used efficiently by those working with them every day, such as:
- Sales teams entering client and sales details
- Marketing departments monitoring response rates
- Accounts teams entering financials
- Customer service personnel compiling feedback
- Engineers reporting on equipment or faults
- Sales and floor staff monitoring promotion uptakes
Whichever teams and individuals are tasked with gathering and entering data on your customers, prospects and business – the importance of carefully checking and accurately adding details must be stressed.
According to Experian’s 2017 global data benchmark report, however, human error is still the main cause of inaccurate data.
TIP: Newer staff members may not have been shown how to input information accurately or how to use data contained in the system. Read the most common data flaws and how to reduce them in the next section: the data quality cycle.
Minimise human error by:
- Avoiding putting constant pressure on data inputters to push information in and out as quickly as possible; under those circumstances, the margin of error is bound to be high.
- Even better, having a robust data management system or CRM that allows you to minimise human error. Eliminating free text fields and forcing users to choose from a predefined list of options allows users to input only certain formats.
- Finally, conducting a professional external audit. This can offer you a more in-depth and thorough review of your data quality, which may be caused from out-of-date data, or result from human error during data entry.
Find out why data auditing ensures your marketing remains effective by reading our blog: why a data audit is necessary.
7. The data quality cycle
Data quality management requires an ongoing repeatable process cycle in which continuous analysis, observation, and improvement lead to overall improvement in the quality of business data and information.
The objective of this cycle is to transition from being reactive to data failures into a proactive business that controls and limits the introduction of bad data into the environment.
As we’ve outlined, the data quality cycle incorporates five fundamental data quality practices:
- Assessment: understand the scope of how poor data quality affects the ways the ways that the business processes run
- Measurement: gather and analyse assessment outputs and concentrate on the data elements that are most critical
- Integration: enforcing data requirements across your business and including data quality processes into the development life cycle
- Improve: use data quality procedures to manage data quality rules, conformance to acceptability thresholds and data entry.
- Manage: review whether data meets the levels of accepted quality, and document the processes for remediation and improvement.
How to discover and limit data flaws
By understanding why manual data entry errors happen and learning ways to avoid them, your company can reduce errors more efficiently.
Introducing common, repeatable processes to your data management will help reduce the frequency and severity of data flaws.
According to Vanguard, there are two common data flaws:
- Transcription errors
These tend to be more common when transcribing words rather than numerical data. These often include typos, repetition and deletion. Transcription is a common problem when employees type quickly — if they hit the wrong key, it’s too easy for them not to notice.
- Transposition errors
Transposition errors tend to happen when staff type numbers rather than words. For example, instead of typing 123, the employee types 132. Again, this is a frequent error for employees who type too quickly to notice mistakes as they go.
TIP: If your employees are responsible for buying marketing data, educate them on the importance of only working with trusted data providers that supply compliant, quality data.
The importance of ongoing education and knowledge
As part of the data quality cycle, your employees routinely collect, buy and utilise marketing data., You must regularly train them on why the quality of this information is so important. The Poor data quality can negatively affect business operations.
This basic understanding of the relevance and importance of their position helps employees feel more responsible for the data they collect or purchase, improving their overall effectiveness and accuracy.
TIP: When selecting a database management system, consider how user-friendly the system will be. Look at the suitability from all users’ perspectives, and consider if you can set different levels of permission for different teams or personnel.
Ensure you have the right software
For ongoing assessment and utilisation of your quality data, confirm that the modules available in the data analysis software meet your business requirements. The functionality or modules you should be looking for include:
- Extract and filter data
- Insight and analysis
- Segmentation and modelling
- Automation
- Forecasting strategy
- Results visualisation
- Campaign planning and ROI management.
Most importantly, make sure that your system has the capacity to grow with your data and your business. You are likely to be adding and checking your data quality all the time, so even though your requirement may not be huge right now, this can grow very quickly if you are gathering and updating your data regularly.
Read more about the importance of choosing and maintaining a robust data management system to keep up with your data quality cycle in our blog.
Related guides and ideas
Explore more ideas
Let us open your mind to new possibilities
Our stories and ideas direct to your inbox