Blogs & ideas
Who should I mail? Insight has the answer
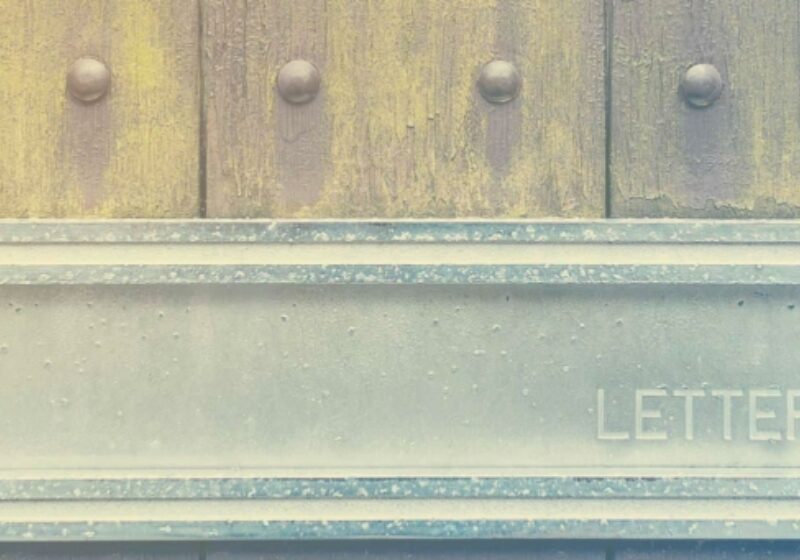
By David Battson 2 min read
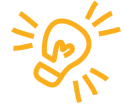
Determining which customers to include in marketing communications is a vital consideration for any campaign. Sending a physical mailing to customers can be expensive, taking into account stationery and print costs and the ever-increasing price of postage.
The first question to answer is what are you trying to achieve? This may seem obvious, but there are many different reasons you may be communicating with your customers, and this will influence the way in which you select the most appropriate audience for the message.
Various techniques are available for selecting and segmenting your mailing data. Testing the different techniques is advisable, to identify the best option for your specific data and campaign activity. These techniques include:
Recency, Frequency, Monetary Value
For companies with a large pool of past customers, the most common method of selecting who to mail is to apply RFV (aka RFM) segmentation. It is a truism that your most recent, frequent and valuable customers are your most engaged. By scoring your customers on these three metrics, you can relatively easily select a subset of your database that is likely to be the most responsive to your mailings.
Predictive Analytics – beyond RFM
Although RFM segmentation is a relatively simple and successful method of selecting a target audience, it is somewhat of a blunt tool compared to some of the more sophisticated approaches now readily available.
Marketing tools like FastStats or statistical packages such as SPSS allow you to compare responders to previous campaigns to your customer base as a whole. This will expose key variables, such as age, gender, geo-demographic factors and elements of previous purchase history, which enables you to define the responsive group by creating an overall propensity score.
Decision Trees
Another approach is to use Decision Trees to find responsive sub segments of your data based on a combination of rules. Rather than just combining together the effect of all the variables, Decision Trees can help expose dependent relationships in your data.
Profit verses Turnover
The next stage goes back to that initial question regarding the aims of the campaign.
There are (too) many times I can recall when, having run predictive models, I have shown a customer compelling evidence of the money they could save by mailing fewer customers, only to be told they are not willing to reduce the projected revenue from a campaign, even if it would result in increased profit.
Consider this example:
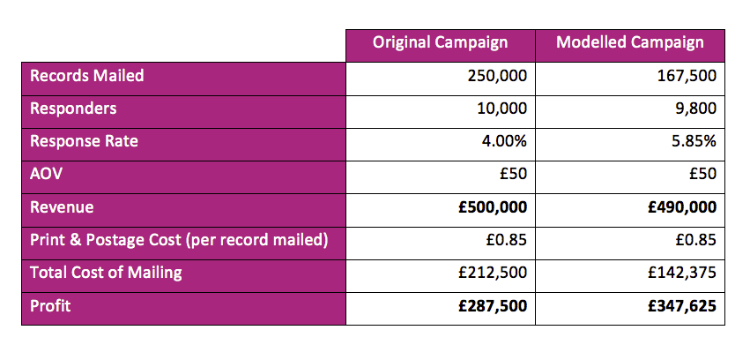
- In this scenario, based on a real-life campaign, sophisticated predictive modelling allows us to identify the most likely responders.
- We can therefore cut the mailing file by 33% whilst still receiving 98% of the responses.
- The suggested Modelled approach results in over £60,000 more profit than the original mailing plan.
Surprisingly, the client almost rejected the approach because it would mean 200 would-be responders would not have received the campaign. It was only when I broke the figures down to the point of explaining that this would mean effectively paying £300 for each additional responder (who would in turn only spend £50), that the client went with using this model.
The other thing to remember is that, in the scenario I have described, the additional profit all comes from savings on print and postage. Those costs come directly from the marketing budget, and could therefore be reinvested in additional marketing activity. Based on these figures you could mail another 70,000 of your high propensity customers.
Related blogs and ideas
Explore more ideas
Let us open your mind to new possibilities
Our stories and ideas direct to your inbox